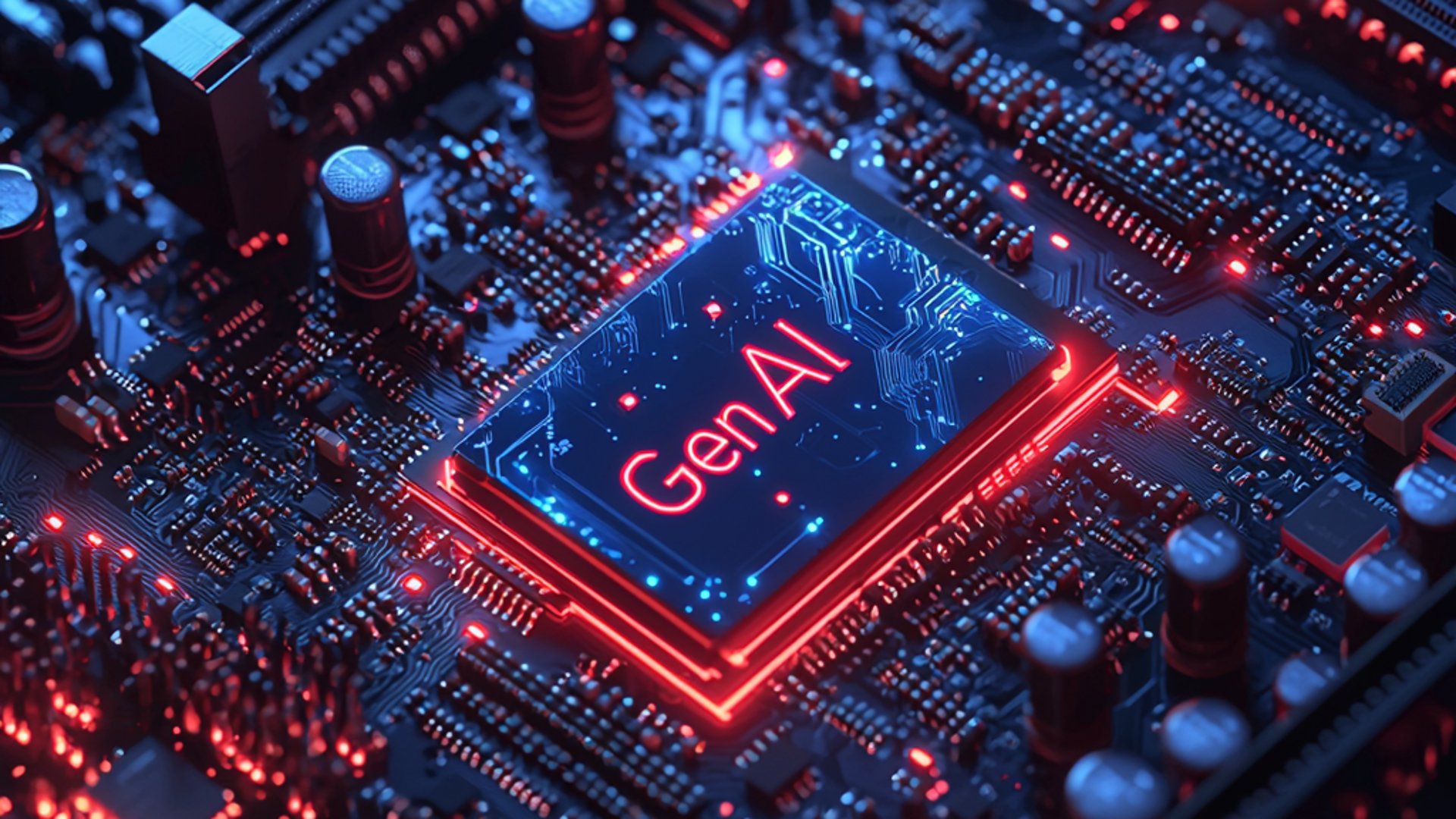
The Transformative Potential of Generative AI in Radiology Workflows
By: James Conyers
September 8, 2024
As AI technology, especially generative AI, continues to evolve, its potential to enhance diagnostic imaging in healthcare is becoming more obvious. However, while significant focus is placed on detection and triage solutions within radiology, the impact of AI on clinical workflows spans far beyond these specifically focused applications. To unlock the true value of AI in radiology, it is essential to address other critical stages of the clinical workflow that can produce immediate results for radiologists, clinical staff, and ultimately patients. This article explores how generative AI and broader AI solutions can be better integrated within the entire radiology workflow, producing more significant and immediate impacts on efficiency, accuracy, and patient outcomes.
A Narrow Focus: Detection and Triage
The current marketplace is filled with AI solutions aimed at improving detection and triage in radiology. These systems are highly valuable in their specific contexts, such as identifying and prioritizing suspicious findings in imaging, thus improving early diagnosis of conditions like cancer. AI-based detection systems, often using deep learning models, have shown tremendous success in tasks like identifying breast cancer lesions with a precision that sometimes exceeds that of human radiologists.
Despite these advances, detection and triage AI tools only address a small portion of the radiology workflow. The challenges of modern radiology are multifaceted, extending well beyond the image interpretation phase. Errors in reporting, coding, interoperability, and data exchange still plague the workflow, leading to inefficiencies and risks for patients and providers alike.
Limitations in Current AI Solutions
Radiology workflows are involved, involving many stages that AI detection and triage solutions alone do not address. These include patient preparation, imaging acquisition, image analysis, peer review, pre and post processing, quality and assurance, reporting, exchange and communication of results to both patients and referring physicians. At each stage, challenges such as interoperability issues, manual interventions, and outdated technology frameworks increase the risk of error, disrupt the workflow, and reduce efficiency.
Interoperability, in particular, remains a major barrier. Without standardized systems, AI solutions often struggle to communicate effectively across platforms, leading to breaks in data exchange that necessitate manual interventions. Additionally, AI solutions have limited integration options with limited flexibility within the clinical workflow. This leads to additional burden on the radiologist and opens the door for more risk to the provider. Together, this leads to higher risks of misdiagnosis, delays, and administrative errors, none of which are adequately addressed by current detection and triage solutions, because they are simply detection and triage solutions!
Beyond Detection and Triage: Opportunities for Generative AI
Generative AI holds promise in addressing several of the overlooked challenges within the broader radiology workflow. Unlike traditional AI, generative AI can analyze and synthesize large volumes of unstructured data, making it ideal for tackling the administrative and interoperability burdens that weigh down clinical workflows. Below are seven key areas where generative AI could provide transformative solutions:
1. Reducing Reporting Errors
Generative AI can significantly improve the accuracy and efficiency of radiology reporting. For example, AI tools can automatically generate structured reports from free-text clinical notes, reducing transcription errors and ensuring consistency across reports. Additionally, these tools can help flag potential errors in reports, ensuring that discrepancies are caught and corrected before the report is finalized and reaches the patient.
2. Improving Peer Review
Radiologists often engage in peer review to ensure the accuracy and consistency of diagnoses. AI-powered tools can assist by automatically flagging cases for review based on predefined criteria or identifying subtle diagnostic elements that may be missed by human reviewers. By improving the efficiency and objectivity of the peer review process, generative AI can reduce diagnostic discrepancies and enhance overall diagnostic accuracy. Utilizing generative AI coupled with detection and triage solutions, the peer review interruption and burdensome workflow could be drastically revolutionized, if not made extinct altogether.
3. Streamlining Radiology Operations
The industry has been talking about how AI can transform clinical operations by automating mundane tasks like scheduling, billing, and coding, but has not talked a lot about the core clinical operations within the radiology workflow specifically. AI can transform the radiology workflow by enhancing the quality and assurance process, intelligent pre and post processing operations, procedure acquisition validation, exam ordering, and collaboration. Next, with the ability to process large amounts of administrative and clinical data, generative AI can help hospitals and imaging centers reduce operational inefficiencies. For instance, it can automate the classification of medical images for billing purposes, reducing coding errors and streamlining reimbursement processes.
4. Integrating Third-Party AI Solutions
The integration of multiple AI solutions can be challenging due to the lack of interoperability between proprietary systems. Generative AI can help bridge these gaps by standardizing data formats across platforms, enabling seamless communication between different AI systems. This allows healthcare providers to leverage multiple AI tools simultaneously, without worrying about system compatibility.
5. Enhancing Precision Medicine
Generative AI is powerful in its ability to analyze vast datasets and generate insights tailored to individual patients. In radiology, this capability can be used to enhance precision medicine by providing more personalized diagnoses and treatment recommendations. By combining imaging data with genetic, clinical, lifestyle information and like population, AI can help radiologists make more accurate and personalized diagnostic decisions, a the time of interpretation.
6. Real-Time Clinical Decision Support
Generative AI can revolutionize clinical decision-making by providing real-time insights and recommendations directly within the radiologist's workflow. By analyzing imaging data alongside patient histories, analyze detection and triage results based on provider population, clinical guidelines, and even regional-specific treatment protocols, AI tools can bring awareness before an error occurs. This capability enhances the ability to personalize treatment plans, improve diagnostic accuracy, and minimize errors.
7. Universal Ontology Alignment
Generative AI has the potential to solve the critical issue of inconsistent ontologies across healthcare systems by acting as an intermediary layer that ensures universal ontology alignment. Healthcare systems often use different terminologies and coding schemes for clinical data, which leads to communication barriers and potential errors. Generative AI can bridge these gaps by translating and normalizing data from various ontologies in real-time. Generative AI’s capability to act as a semantic translator enables seamless data exchange, ensuring consistent language across systems and reducing errors in clinical decisions.
Immediate ROI and Future Integration
The benefits of applying AI to these critical areas of the radiology workflow extend beyond patient care. Hospitals and clinics that adopt AI in these less visible but essential stages of the workflow can expect to see an immediate return on investment (ROI). The automation of administrative and clinical tasks, the reduction of errors, and the optimization of clinical operations all contribute to increased efficiency, lower costs, and improved patient outcomes.
By first focusing on these foundational improvements, healthcare providers can create a more supportive environment for the broader integration of advanced AI tools, such as detection and triage solutions. A smoother and more efficient workflow will reduce the friction that currently impedes the adoption of AI, increasing the overall ROI and facilitating more comprehensive AI integration across the diagnostic imaging process.
Conclusion
While detection and triage solutions are important, they represent just one piece of the larger puzzle in radiology workflows. By expanding the focus of AI implementation to other stages of the clinical workflow, such as reporting, peer review, and operations management, healthcare providers can significantly enhance the efficiency and accuracy of diagnostic imaging. In addition, providers can begin to address the challenges they face with capacity, erosion of margins in diagnostic imaging, and the continuing imaging volume growth. As generative AI continues to develop, its potential to revolutionize not just radiology, but healthcare as a whole, becomes increasingly apparent. The key to unlocking this potential lies in addressing the broader spectrum of challenges that the radiology workflow faces on a daily basis.
This article serves as the starting point for a deeper exploration of how AI can truly transform radiology from the inside out. My goal is to contribute anyway I can to the continued evolution of diagnostic imaging, hopefully to spike creative out-of-the-box thinking and get us thinking about things differently. The potential that exists is outstanding, whether we capitalize on it sooner rather than later will be up to us. In the end, our focus and dedication should be on advancing healthcare and helping patients.
Article 2-1
James Conyers, Health Tech Innovator